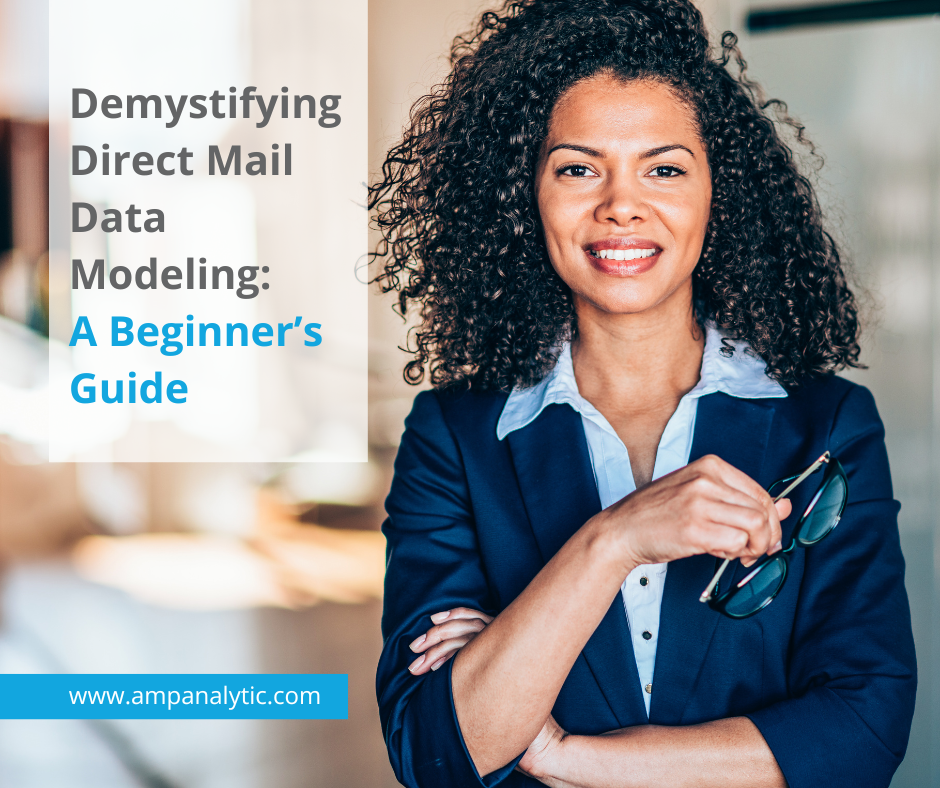
Even in the digital age, direct mail remains a powerful tool for reaching potential customers, especially when combined with data modeling techniques. In this beginner’s guide, we will walk through direct mail data modeling and the benefits of using it in your campaigns.
Key Components of Direct Mail Data Modeling
Data Collection:
The first step to building out a model is collecting customer data. This includes first and last names, postal addresses, past purchase behavior, etc. The more comprehensive the data, the better the model will be able to predict response rates.
The most important information to collect is names and postal addresses. If your database consists of names and emails or postal addresses only, data scientists can append missing information to the dataset. Match rates will vary depending on the information you do have available as well as the quality of the data.
Data Hygiene and Preparation:
Raw data often contains errors, inconsistencies, and missing values. Data hygiene involves multiple steps to identify and rectify these errors. Data scientists will align formatting, update addresses so they match what the United States Post Office has on file, suppress bad records from the list, merge duplicate records, split values as needed, etc.
This step is critical to the success of the model. If you put unclean data into a model, you will receive skewed results. The cleaner your data list is the more accurate your results will be.
Variable Selection
After the data has been cleaned and prepared, data scientists will then append demographic, lifestyle, and geographic data to the file. This offers layers of information about your customers. However, you do not need every variable to build the model, so data scientists will examine what data points are the most valuable for predicting response rates for your unique customer database. They will remove others that are irrelevant or do not hold any weight in predictability.
Model Building
Data scientists will then use their variable selections to create a model. This model analyzes the customer data to identify patterns and predict the likelihood of someone responding to your offer. Once the model has been established, you can use it to identify prospects who are most likely to buy from you.
Validation & Testing
Data models have to be able to accommodate for all sorts of conditions and variations, so data scientists will test the model performance in order to validate the results and ensure its accuracy. These tests are validating the model’s ability to predict results and looking for any potential issues that might deliver inaccurate results.
Benefits of Direct Mail Data Modeling
Improved Targeting
Direct mail data modeling identifies individuals who are more likely to respond positively to a direct mail campaign. Marketers can select their best prospects and target their efforts more effectively, resulting in higher response rates and ROI.
Cost Efficiency
By targeting the people who are most likely to be interested in the offer, marketers can minimize their marketing waste and optimize their budgets. They can confidently spend their resources on their most promising prospects.
Personalization
Direct mail data modeling allows marketers to target known individuals, so they can personalize their mail pieces. With the modeling information, they can take into consideration the recipient attributes, preferences, past engagements, etc. This level of customization helps increase engagement and drive conversion rates.
Iterative Improvement
By targeting known individuals, data scientists can identify true responses and use that information to continuously refine the model. This iterative process generates ongoing improvements in campaign effectiveness over time.
Conclusion
Direct mail data modeling creates a powerful approach to enhancing the effectiveness of direct mail campaigns. By leveraging data analytics and predictive modeling techniques, data scientists can identify and target the most promising prospects. This creates higher response rates, increased ROI, and a more personalized customer experience.
If you would like to learn more about data modeling or see if it’s a good fit for your business, give us a call. Our team would be happy to walk you through the process.